How to develop increasingly complex AI systems with a smaller amount of data… “It’s not whoever has the best algorithm that wins, it’s whoever has the most quality data.”
The words of artificial intelligence researcher Jean-Claude Heudin illustrate the essential nature of data within AI algorithms1. However, data collection, processing and storage are time-consuming and costly processes.
Machine learning systems and their objectives are increasingly difficult to characterize as they become more complex. The challenge today is to design AI systems that require less data while guaranteeing a certain robustness within the envisaged field of use. This is why researchers and engineers are working to develop so-called frugal artificial intelligence systems that require less data and less computing power to build them.
The climate issue is crucial today because of the environmental impact of data centers. It is estimated that the electricity consumption of data centers doubles every four years. And this is without considering the environmental cost necessary for their manufacture. Data centers could consume 10% of the world’s electricity production by 2030, compared to only 3% today2. However, companies are, above all, looking for frugal AI in order to overcome the lack of data available to them.
Indeed, in some applications it is not necessarily possible to obtain a sufficient amount of data. For example, in the military domain, AI systems are trained to recognize vehicles. However, for obvious reasons, some nations will not provide a complete database containing images of their own vehicles. But defense is not the only sector where frugal AI is needed. The medical field could, in the future, benefit from personalized medicine capabilities to each patient. Indeed, since each disease and patient is unique, an algorithm that adapts to each patient could help them better manage their pain or recovery. Here frugal learning is essential because the system learns from only one source of data: the patient himself.
Frugal AI is still in its infancy and is today an active field of research. It is still difficult to train a model from a relatively small amount of data, or even from a single instance (one shot learning). However, there are methods to overcome or bypass this lack of data.
Transfer learning
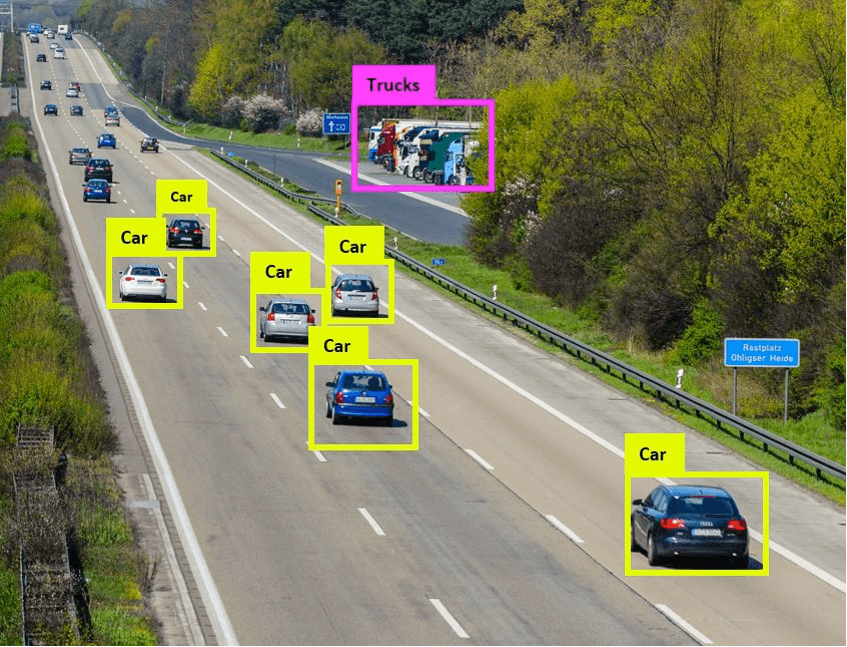
This method consists in reusing an existing AI system that has started to learn from a sufficient dataset. The idea is to start with an AI that has learned from data that is somewhat similar to the missing data. For example, the objective is no longer to identify cars but armored vehicles. At first, the AI system is trained to recognize cars, but without being very efficient. The goal is to make it learn to recognize only features that are common or relatively close to those that the final system will have to learn. Data scientists learn the second system by injecting data from the first one and then completing its learning based on the few data they have.
Data generation and data augmentation
Data generation consists in using a virtual environment to generate as close as possible to the conditions of a real environment. The objective is to generate the missing data for training directly from this simulation environment.
So, let’s go back to our example of an AI system to recognize an armored vehicle. Some specialized companies will build a faithful reproduction of the vehicle in 3D and then generate the images of the vehicle in the appropriate scenarios. The idea is that it becomes possible to obtain an almost infinite amount of data containing images of the object to be detected in multiple scenarios. The main challenge of this approach is the passage in real condition, where the performances of the system are not necessarily maintained.
On the one hand, it is difficult to reproduce a real environment in a totally realistic way. On the other hand, there is a risk that the AI learns more about the biases of the modeling than about the modeled object. If data cannot be generated ex nihilo, it is also possible to generate new data from existing data with data augmentation techniques. To do this, this technique declines by disturbing an existing dataset (rotation, addition of blur, image degradation). It is also possible to link data generation and data augmentation in order to make a dataset more complete. However, data augmentation is not a miracle solution. Indeed, it does not allow to extend the domain of use of an AI system, but only to reinforce its robustness in an already covered environment.
The frugal AI seems to have a bright future ahead of it because of the proactive policy of Europe that it generates. Through several Horizon Europe and EDIDP projects, the 27 Member States are seeking to reduce the digital footprint of AI, but also indirectly to increase their independence and digital sovereignty in the face of GAFA and BATX3.